This is the ninth post in a series about improving human-bot relations in customer service. See my page to get caught up.
Over the past several posts, we’ve looked at how the human-powered side of our customer service operations could dramatically benefit from “computational thinking.”[1]
The next series will look at the flip side: why chatbots should be “managed” more like people. But before moving on, I’d like to recap what we’ve discussed so far.
- A Human Response to the Rise of Chatbots. To kick off this series, I cited the investment dollars flowing into AI-powered customer service chatbots as a proxy for innovation occurring within the space. The sheer number of investments shows that we’re mad about bots. While this is destined to be a good thing, I opined that, along the way, we seem to have forgotten about the human-powered side of customer service. We have become obsessed with chatbots because doing customer service is hard and we see bots as a possible fix.
- The Sociopathic System: Why some customer service programs consistently let us down. This next post exposed a crucial risk: ignoring the human side of the equation, as we seem to be doing, risks building our AI-systems over-the-top of an incomplete or even faulty foundation. Most failures are caused not by ignoble agents but by systems, policies, and priorities that set up customer service agents for failure.
- Using software to be more human: 5 ways computational thinking will revolutionize human-powered customer service. If the first two posts were approximately correct, we’re ignoring the human side of customer service at our own peril and installing bot systems over-the-top of an incomplete foundation. To combat this, I posited 5 ways we can use “computational thinking” to improve human-powered support and set the stage for the next 5 posts.
- Stick to your priorities without becoming a robot: improve organizational focus in customer service. Here I contrasted perfectly focused bots with easily distractible humans and recommended that we encode our business priorities into the fabric of our execution and recognition systems to engineer a payoff for on-track work.
- Stop taking mental shortcuts: improve resource allocation in customer service. This post reminded us of the heuristics we often use to simplify difficult problems such as detailed case routing. I claimed that, with today’s technology, mental shortcuts are no longer necessary. We can improve results by attacking routing (and similar complexity) head-on.
- You’re gonna need a bigger boat: improve scalability in customer service. A third improvement made possible with computational thinking is the demand-supply problem of customer service capacity. Instead of using traditional, expensive, and sometimes painful headcount-based maneuvers, I described a more modern, technology-enabled approach to dynamically match capacity to demand.
- Taking a punch: improve resiliency in customer service. In this post, the lesson learned from computational thinking is to create redundancy in your support network and use technology to quickly adjust when there is a demand or capacity shock to the system. If the pandemic has taught us anything, it is that there is a real price to be paid for being unprepared.
- The “Case Economy:” decentralizing to improve decision-making in customer service. Scalable computer systems, especially networks with many nodes or endpoints, have mastered the balance between central and local rule systems. This post affirms the application of a similar approach to push decision-making, with the aid of technology, closer to the end customer.
At the individual level, it’s still about people helping people, with our glorious range of differences and personalities. But this series of posts has asserted that human-powered support should be thought of as a system: one that has been thoughtfully designed to smartly tap our people resources and let agents play to their strengths. The time for oversimplification, self-limiting, and centralization has past.
By letting our networks learn and adapt the way a software program (such as a chatbot) does, we will improve our focus, resource allocation, scalability, resiliency, and decision-optimization.
Bots won’t make us better people, but they might make our people better.
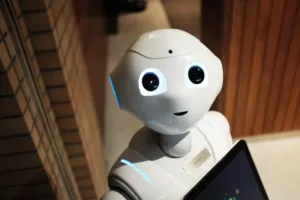
Photo: Alex Knight via Pexels
Up next: We’ve discussed how humans can learn from bots. Next, let’s explore the idea of “managing” chatbots more like we do people.
[1] https://www.nytimes.com/2017/04/04/education/edlife/teaching-students-computer-code.html
Related Articles
Want more? Here are some other blog posts, topics and articles you might be interested in.